Data APIs with RAW
RAW is the modern platform for building and hosting data services.
Aggregate, join, and transform data from anywhere in real-time.
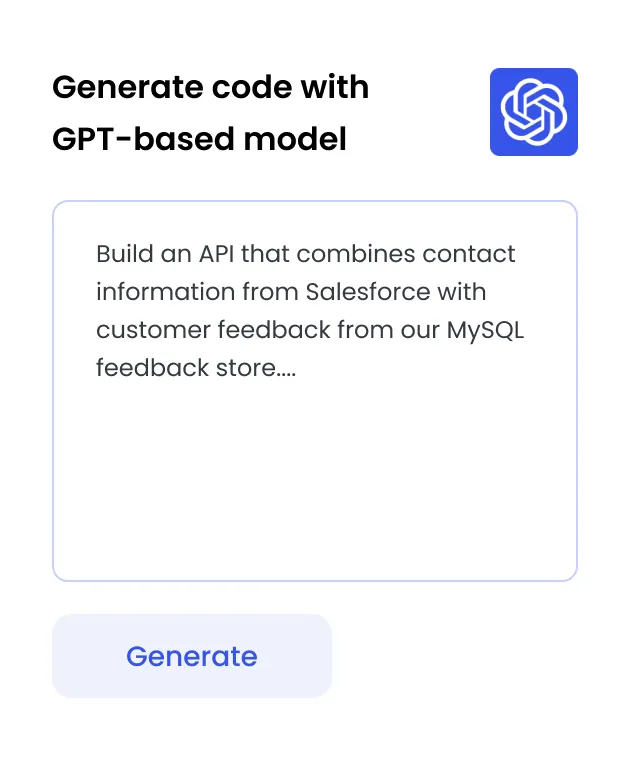


Build data services to…
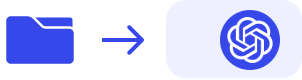
Feed data to LLMs
Create and host APIs that feed data into AI systems and integrate with services like ChatGPT plugins or GPT personas.
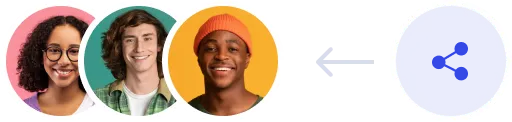
Share data with customers
Turn-key solution to modernize and monetize your data delivery with a modern and fully-managed data hub.

Real-time Data
Aggregate and integrate data in real-time from S3, data lakes, cloud and on-premises databases and serve as APIs.
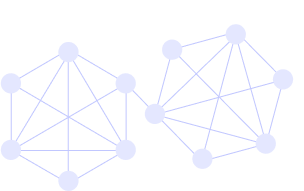
Create a data mesh
Turn-key solution to modernize and monetize your data delivery with a modern and fully-managed data hub.
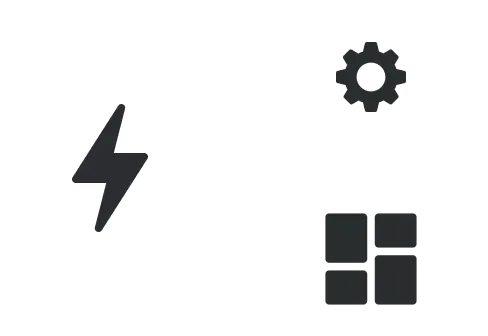
Fast prototyping
Quickly build data services using data from multiple sources. Quickly deploy, test and iterate your data architectures.
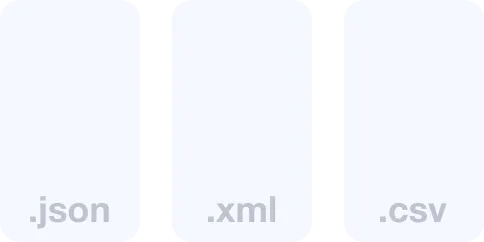
Mock data
Speed up your development with powerful mock APIs for JSON, XML, CSV and other formats.
Spend less time building and more time innovating!
Build fast with AI
Effortlessly build and host data services, whether for internal use like internal APIs or dashboards or for external use like making your data available to ChatGPT.
Scales with your needs
RAW is an all-in-one, easy-to-use platform that scales with your needs and with your team, with exceptional time-to-value while keeping it simple and keeping you agile.
Traceable and Secure
Build, host, and share your data services confidently, thanks to a type-safe programming language, secure development environment, and a rock-solid platform with full auditing and traceability.
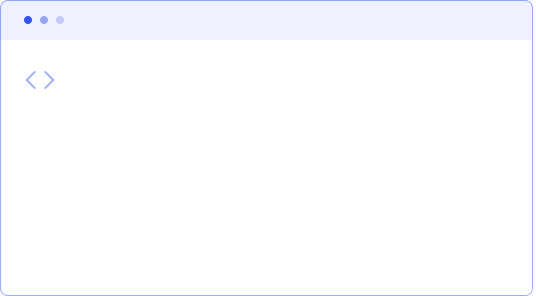
Build
Low code development.
Build data services using Snapi, a low-code language that allows you seamlessly explore data, join and transform it in real-time from any source.
Built-in IDE.
Instantly start building and testing with a fully-integrated IDE that lets you code, test and experiment in an easy-to-use environment.
Data Connectors.
Out-of-the-box support for the most widely-used databases, popular data formats and data lakes. No dependencies needed, no installation required!
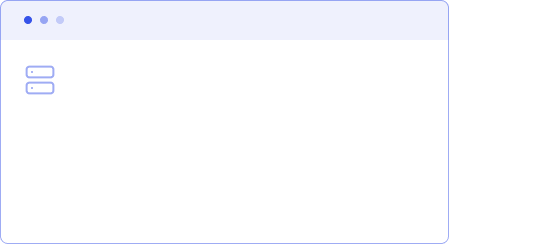
Host
Push to Deploy.
Serve data as APIs with a single click or read them from a GitHub repository. Set security rules and deploy services in production in seconds.
Integrated Testing.
Code in an isolated GitHub branch to test your new services separately from production. Test results are reported automatically with GitHub pull request integration. Create new API versions safely and migrate all endpoints atomically.
Built-in API Management.
Enforce usage policies, access control, collects statistics, monitor performance. Also seamlessly integrates with existing API management tools.
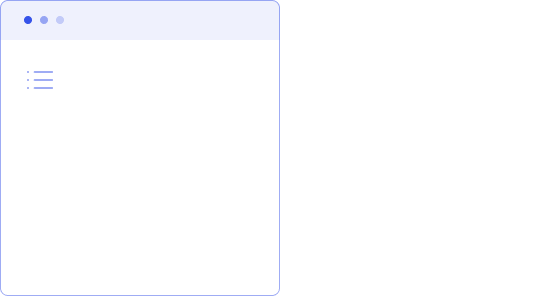
Share
Built-in Data Catalog.
Includes an OpenAPI-compatible catalog that is automatically created and maintained from your API definitions and code. Also supports exporting metadata to existing data catalogs.
Secure Platform.
Offers vertically-integrated language and platform security, user and API key management, monitoring, and auditing capabilities.
Fine-grained Permissions.
Control who sees what with fine-grained control. Hide, rewrite or otherwise obfuscate sensitive information based on the consumer of the data.
Explore how people use RAW to automate API building
Built by researchers, committed to open source
RAW is powered by Snapi, our home-grown data analysis language built from the ground up for real-time data analysis. Snapi has a rich built-in library with ready-to-use connectors to the most common data formats, data lakes, and databases.